efi robotics
engineering for intelligent robotics
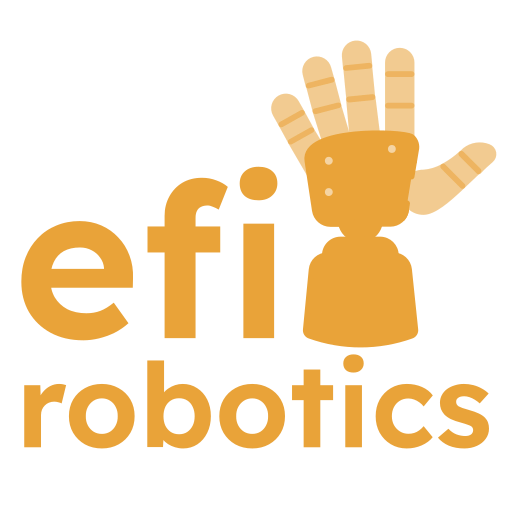
Group based in the School of Engineering Mathematics and Technology and led by Dr Efi Psomopoulou, Assistant Professor at the University of Bristol and Bristol Robotics Laboratory. Part of the Dexterous Robotics Theme.
research areas
We aim to create dexterous and safe robotic physical interactions with the environment or humans in unstructured scenarios by combining control methods and machine learning techniques.
- Stable object grasping and in-hand manipulation.
- Human-inspired human-robot object handover strategies.
- Control of flexible joint robots with variable elasticity.
- Non model-based control of robotic systems with prescribed performance guarantees.
- Bilateral teleoperation with haptic feedback.
latest posts
May 08, 2025 | Postdoctoral position available |
---|---|
Feb 20, 2025 | ARIA project awarded! |
Sep 10, 2024 | MultiTip project awarded! |